Text Sentiment
Text Sentiment Analysis
Text sentiment analysis enables companies to harness the power of machine learning algorithms to efficiently analyze text data and extract meaningful insights. Businesses can leverage this technology to efficiently analyze user reviews, social media comments and feedback to understand customer sentiment towards their offerings.
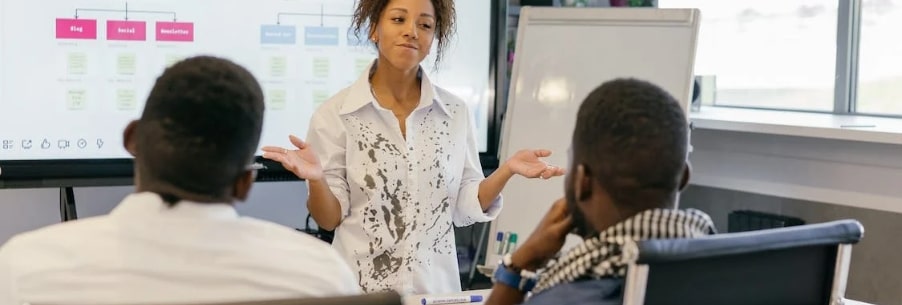
Text Sentiment Analysis: Introduction
According to the National Language Processing Research Laboratory (NLPRL), sentiment analysis delivers an accuracy rate of 70% to 80% in identifying positive and negative opinions. As technology continues to evolve, this precision will only get better, and various industries are now utilizing this technology to boost productivity.
Here are some examples of how text sentiment analysis is being leveraged to monitor user sentiments:
- Customer Reviews: An online retailer can use sentiment analysis to evaluate customer reviews for product insights. This allows them to quickly identify any issues and take action to improve customer satisfaction.
- Social Media Monitoring: Brands can track comments and mentions of their name on social media using sentiment analysis. By analyzing the overall sentiment of responses on posts (positive, negative, or neutral), they can identify trends or issues and proactively address them.
- Chatbot Conversations: Customer service teams leverage sentiment analysis to evaluate the effectiveness of chatbots' responses. By identifying areas for improvement, companies are able to provide better customer support and enhance customer satisfaction.
Overall, text sentiment analysis is a valuable tool that enables businesses to gain insights into their customers' opinions and improve their products and services accordingly.
Types of Text Sentiment Analysis
Graded Sentiment Analysis
- Very positive
- Positive
- Neutral
- Negative
- Very negative
Emotion detection sentiment analysis
Aspect-based Sentiment Analysis
Multilingual sentiment analysis
Virtual Reality
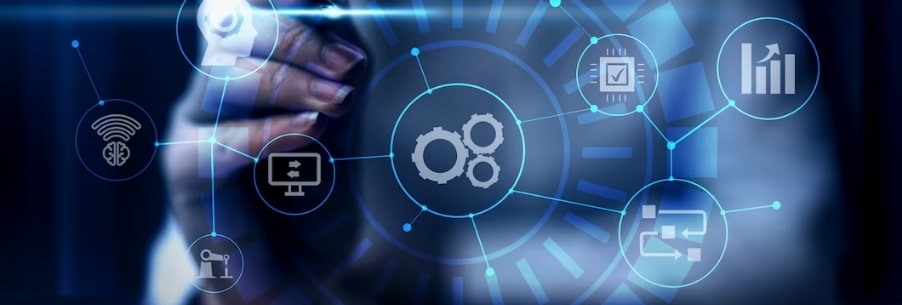
How Text Sentiment Analysis Tool Work?
- Data Collection: Text data is collected from various sources, such as social media platforms, customer review sites or chat logs.
- Preprocessing: In this step, the text data is cleaned and prepared for analysis, which involves removing stop words (common words like "the" and "and"), stemming (reducing words to their root form) and tokenization (splitting text into individual words or phrases).
- Feature Extraction: The text is converted into a numerical representation that can be used by the machine learning algorithm. This may involve techniques like bag-of-words (counting the frequency of each word) or word embeddings (representing words as vectors in a high-dimensional space).
- Model Training: The machine learning algorithm is trained on a labelled dataset (text data that has already been classified into sentiment categories).
- Prediction: At last the trained model is used to predict the sentiment of new, unlabeled text data.
For example, let's say we want to analyze the sentiment of customer reviews for a restaurant. We would collect the text data (reviews), preprocess it by removing stop words and stemming the words, extract features using bag-of-words, train a machine learning model on a labelled dataset of reviews and then use the model to predict the sentiment of new reviews.
Use and Applications of Text Sentiment Analysis
Customer Feedback & Support
Brand research
Product analytics
Sales enablement
Challenges and Concerns
Accuracy
Bias
Context
Request a Live Demo!
See how Facial Coding can
help your business
Book your slot now!
Other technologies similar to facial coding
Latest from our Resources
How AI in Marketing Research Transforming the Industry
In these day’s fast-paced  enterprise environment, staying before hand of the competition is making properly-knowledgeable alternatives based totally on accurate, up to date information. Traditional advertising research strategies, at the same time
Read MoreWhat Are Online Focus Groups and How Do They Work?
In the age of virtual transformation, corporations want innovative techniques to recognize their clients and make information-driven picks.
Read nowWhat Is Facial Coding and How Does It Work?
Facial coding, additionally known as facial motion coding, is the systematic evaluation of human facial expressions to interpret feelings
Read now