Speech Transcription
Speech Transcription Technology
Companies today transcend geographical or cultural borders, so customers might face issues understanding certain dialects, terminologies, or other speech elements while speaking to business representatives. In addition, there can be mismatches in understanding or retaining parts of the conversation sometimes. Having speech transcription support can be particularly helpful here in recording the exact conversation in textual format to offer improved transparency and customer experience.
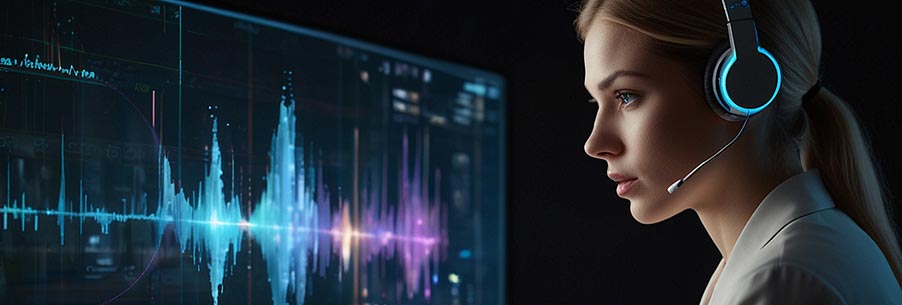
Speech Transcription: Introduction
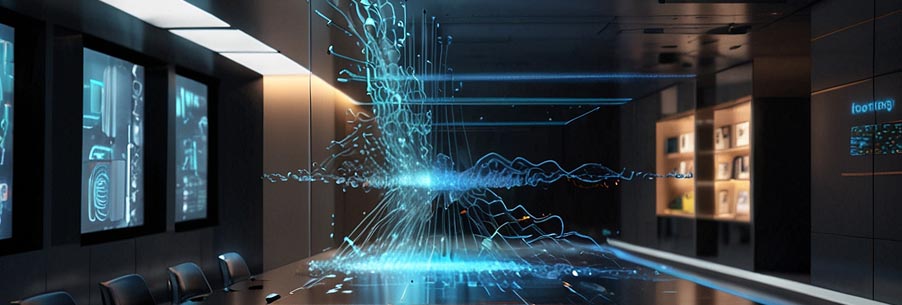
How does speech transcription work?
The first steps in converting speech to text include digitizing the sound and converting the audio data into a format that a deep learning model can handle. The processed audio is then transformed into spectrograms that visually represent sound frequencies, making it possible to differentiate between sound elements and their harmonic structure. The spectrograms facilitate audio classification, analysis, and representation of audio data.
Subsequently, the sound is classified into distinct categories, and the deep learning model is trained on these categories. It allows the model to predict the class to which a particular sound clip belongs. Hence, a speech-to-text model utilizes input features of a sound to correlate with target labels, which comprise spoken audio clips and their corresponding text transcripts.
In simple terms, speech-to-text software listens and records spoken audio and then produces a transcript that aims to be as accurate as possible. A computer program or deep learning model with linguistic algorithms is employed to achieve this, which works with Unicode, the global software standard for text processing. A complex deep learning model based on various neural networks is utilized to convert speech to text through the following steps:
- Analog to Digital Conversion:
Speech-to-text models pick up the vibrations produced by human speech, which are technically analog signals. An analog-to-digital converter converts them into a digital format. - Filtering:
The digitized sounds are in the form of an audio file, which is analyzed comprehensively and filtered to identify relevant sounds that can be transcribed. - Segmentation:
The sounds are segmented based on phonemes, the linguistic units that distinguish one word from another. These units are compared to segmented words in the input audio to predict possible transcriptions. - Character Integration:
A mathematical model consisting of various combinations of words, phrases, and sentences is used to integrate the phonemes into coherent phrases or segments. - Final Transcript:
The most probable transcript is generated based on deep learning predictive modeling, and the built-in dictation capabilities of the device produce a computer-based demand for transcription.
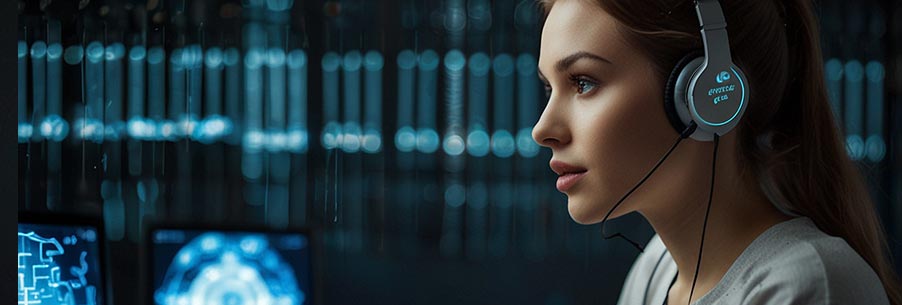
Speech transcription with AI: The current landscape
AI, ML, and NLP are three important buzzwords of modern speech recognition technologies. Although these terms are often used interchangeably, they have different meanings. AI refers to the vast field of computer science dedicated to creating more intelligent software that can solve problems like humans. ML, on the other hand, is a subfield within AI that focuses on using statistical modeling and vast amounts of relevant data to teach computers to perform complex tasks, such as speech-to-text. Finally, NLP is a branch of AI that trains computers to understand human speech and text, enabling them to interact with humans using this knowledge.
While basic text-to-speech AI can convert speech to text, advanced tasks like voice-based search and virtual assistants like Siri require NLP to enable the AI to analyze data and deliver accurate results that match the user's needs.
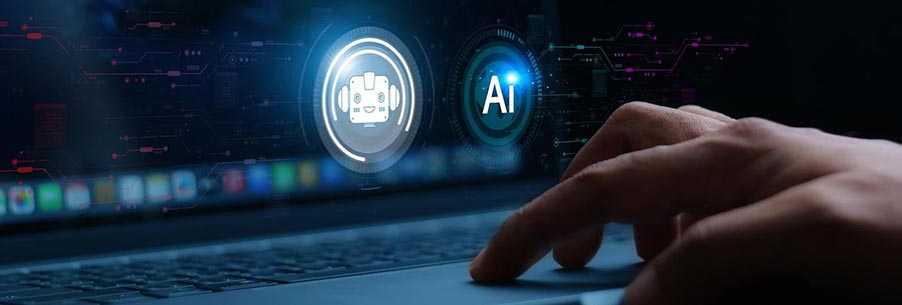
AI that converts speech to text
Google Speech-to-Text is an API service that uses machine learning to convert audio to text. It can transcribe real-time streaming or pre-recorded audio and supports multiple languages and dialects. It is widely used for applications such as speech-enabled customer service, real-time closed captioning, and transcription of recorded meetings and interviews.
Amazon Transcribe is another cloud-based speech recognition service that automatically converts speech to text. It can handle a wide range of audio formats and can recognize different speakers in a conversation. It also provides a confidence score for each transcription, making it easier to identify areas that require human review.
Microsoft Azure Speech-to-Text is a cloud-based speech recognition service that supports several languages and can handle real-time streaming and pre-recorded audio. It also includes features such as speaker recognition and customization options to improve accuracy for specific industries and domains.
Apple's Siri is a virtual assistant that utilizes speech recognition to understand and respond to user requests. It can perform a wide range of tasks, such as sending messages, making calls, and playing music, all through voice commands. Siri is built into Apple devices such as iPhones, iPads, and Macs and constantly improves through machine learning and natural language processing.
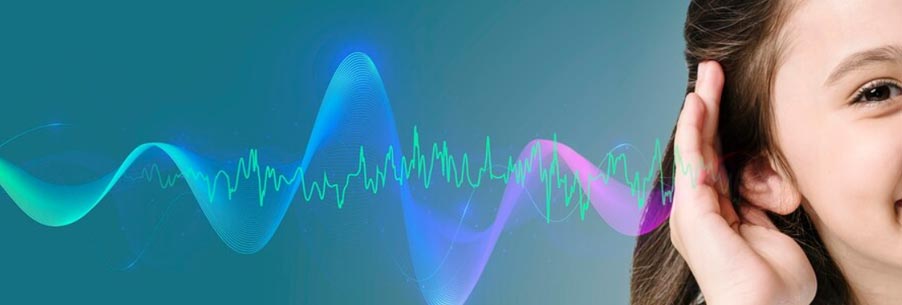
Speech recognition transcriptionists: A new role on offer?
Speech recognition transcriptionists may work in the healthcare, legal, and media industries. They may transcribe medical dictation for doctors and other healthcare professionals in the healthcare industry. In the legal industry, they may transcribe court proceedings and depositions. In the media industry, they may transcribe interviews, speeches, and other spoken content for journalists and broadcasters.
Speech recognition transcriptionists need to have excellent listening and typing skills and knowledge of grammar and punctuation. They should also have specialized expertise in their industry, such as medical terminology or legal jargon. Some employers may require certification or formal training in speech recognition technology or transcription.
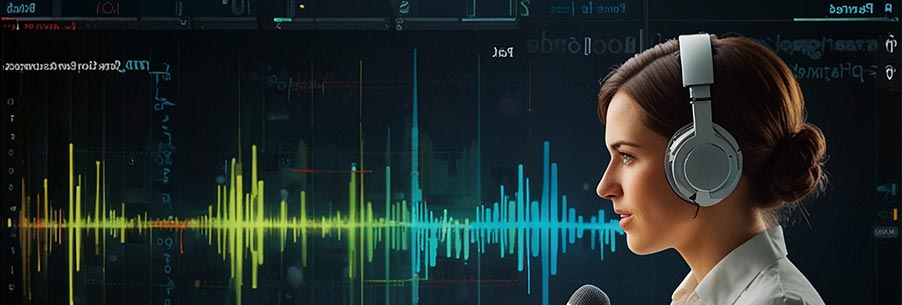
Speech recognition vs. speech transcription - are they the same?
Transcription and ASR are two different processes with unique characteristics. Transcription does not direct the call flow based on what the caller says. It records an audio file and attempts to interpret it into written text without predetermined grammar or keywords. The transcription accuracy depends on the recording quality, with clear and well-recorded files having a higher confidence level than those with poor sound quality.
Transcriptions can be categorized based on the engine's confidence level, which can be high, medium, or low. While a clear recording tends to fall into the high confidence bucket, a recording with poor sound quality falls into the lower confidence bucket. It is often used for open-ended questions, such as customer feedback surveys. Although human transcription tends to be more accurate, it is more expensive and takes longer than computer transcription.
ASR differs from transcription in several ways. Firstly, ASR makes speech a valid form of data input, allowing end-users to influence call flow and redirect the caller based on what they've said. Secondly, ASR is programmable and relies on keywords or expected responses, reducing the number of possible answers and making speech processing faster and easier. To control speech input, speech recognition engines use grammars, which are collections of possible responses and can recognize the answer to a yes/no question, for example, by including words like "yes," "yeah," and "yup" in the grammar. However, an error may occur if the end-user says something outside the grammar. ASR is commonly used for capturing alpha-numeric data such as names or addresses and for hands-free calling, mainly if end-users frequently make calls while on the go.
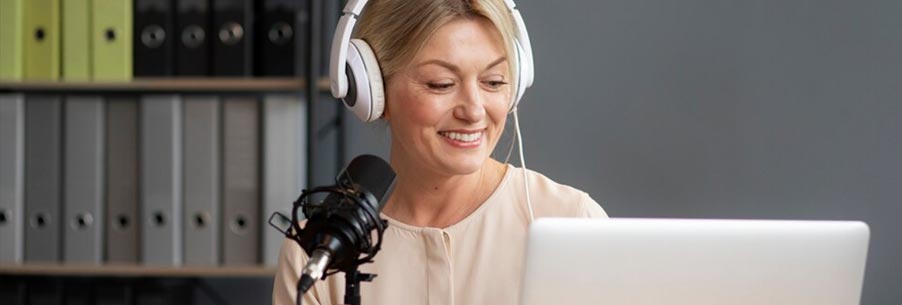
Live Transcription: The accessibility feature with Video Conference platforms
How speech transcription can be used for market research
Better administration
Reduce overhead costs
Add more value to market research:
Centralized database
Accuracy in data extraction
How speech transcription helps in sales enablement
Improves active listening
Improve agent appreciation
Effective sales team coaching
Offer better customer experience
Ethical concerns
Limited application
Limited accuracy
Direct interviews analysis
Request a Live Demo!
See how Facial Coding can
help your business
Book your slot now!
Other technologies similar to facial coding
Latest from our Resources
AI-Driven Innovation: Redefining Best Practices in UI/UX Testing
Introduction User interface and user experience testing (UI/UX testing) are two principal factors that behave as momentum for software development process providing digital solutions with top notch user friendliness and high level of users satisfaction. As a
Read MoreAI-Assisted UI/UX Testing: A Game-Changer in Product Development
Introduction: As users adopt digital products, delivering a flawless and remarkable user experience becomes one of the most critical
Read nowFacial Coding AI: Everything you need to know
This questions perhaps has lingered in our minds several times; why can we not easily hide our emotions as we do with our physical
Read now